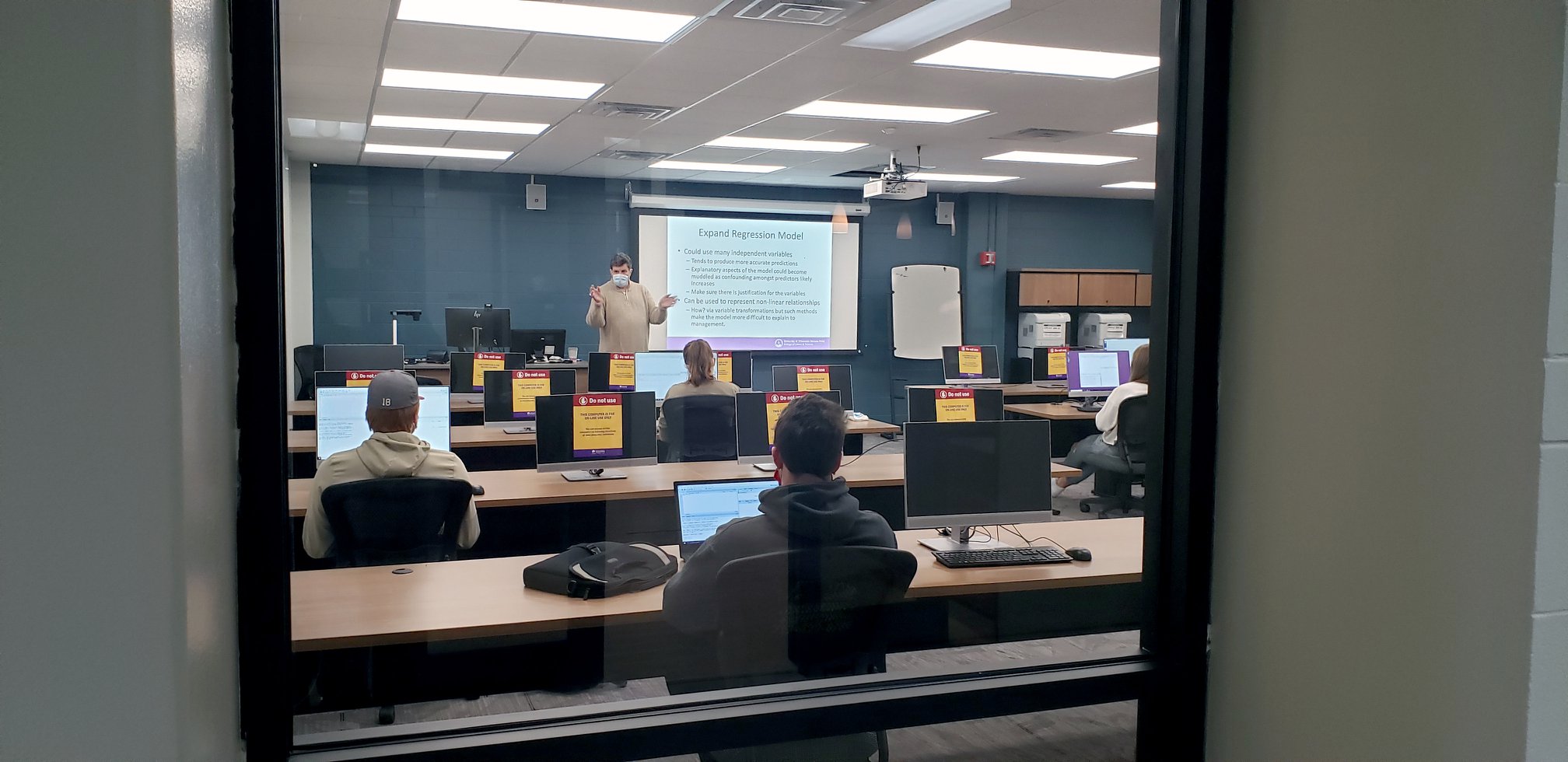
The following article was originally published in the Center for Business and Economic Insight Fall 2020 Report.

by Kurt Pflughoeft, Ph.D.
Sentry Endowed Chair of Computational Analytics; Director of the Center for Data Analytics
Data analytics is all the buzz at many corporations subsuming areas such as business analytics and business intelligence. The goals of data analytics are broad, ranging from knowledge discovery to automated decision-making. The impact of this field has led to many benefits such as increased cross- and up-selling as well as identifying new business opportunities.
Now, if for some reason, you are a bit skeptical about this field, you may ask, “Isn’t data analytics just a rebranding of past quantitative approaches like statistics, operations research or computer science?” The answer is: “Not quite.” Although these fields provide many contributions to data analytics, there have been great advances with regards to algorithms, software and hardware that have led to synergies which are leveraged by data analysts.
Data analysts have a “can-do” attitude to arrive at solutions even if an unconventional approach may be needed. In fact, a few short years ago, the American Statistical Association, was worried that applied statistics was being eclipsed by fields like data analytics. The association made the following recommendations to its members:
- Gain a deep understanding of the product/service that you are supporting.
- Be more focused on predictions rather than merely inferring relationships.
- Be willing to tackle large and messy problems.
- Create more partnerships between the statistics and data analytic communities.
These recommendations were patterned upon the successes of data analytics. For example, large and messy problems may be characterized by unsatisfied assumptions or by big, dirty and unstructured data. Many statisticians had not wanted to deal with such issues.
Likewise, managers don’t want to hear about excuses why a problem is too difficult to address; they want insights to guide their decision making. Solutions need not be optimal but “good-enough” solutions can be quite helpful.
While working at a market research (MR) firm, I experienced some of the upheavals caused by data analytics. Most of our newer competitors were no longer other MR firms but rather data analytics firms. This observation was also evident from the confusion surrounding the revered Honomichl list of MR firms. Is a data analytics firm that tackles many MR problems an MR firm? Probably.
An early example of the use of data analytics for an unconventional solution was the determination of audience share for TV programs. MR firms used many different techniques ranging from TV diaries to monitoring devices. The “audience share world” was reset when a professor simply analyzed tweets to come up with the same results. Tweets are now a standard way to determine, in part, audience share.
A more recent example is the prediction of customer attrition by MR firms that measure satisfaction. Their method to determine potential defections relied on “Hot Alerts.” A “Hot Alert” identifies customers who may have given the client a low score on a key question.
Although “Hot Alerts” have some benefits, they may be too late for intervention and they only address surveyed customers. Data analysts brought much more horsepower to identify potential defectors early on. For example, a TV broadcast company issued a request for proposal (RFP) to help with this task. The data analysts requested many more variables including call center contacts/notes, web site navigation, services bundles, customer viewing habits, customer age and location to name a few.
By using sophisticated modeling techniques, such as Random Forests, and big data ecosystems like Hadoop or Spark, data analysts could leverage all that data and make accurate predictions. In fact, the TV broadcast company was quite surprised how good the model was. It substantially outperformed their long-established internal model.
In fact, you can see some of these competitions yourself on websites like Kaggle. An organization may post a particular problem and a dataset, often anonymized, for competition. The person or team that submits the best solution may win a prize. Some more recent Kaggle competitions include:
- Two Sigma: Using the contents of newspaper articles to predict stock market performance.
- Radboud University Medical Center: Detecting prostrate cancer from images of tissue samples.
- NFL: Analyzing video to detect helmet impacts that occur on the field
- Online Retailer (anonymous): Identifying high-valued customers that may benefit from a loyalty program.
Data analysts perform four broad categories of analysis which are briefly mentioned below.
Descriptive Analytics help summarize the past and current situations either visually or numerically. A common task might be to develop a key performance indicator (KPI) to help the firm keep track of successes or failures.
Diagnostic Analytics determine why things have happened. For example, an anomaly may have been identified by the descriptive analysis and now its root cause needs to be identified.
Predictive Analytics is all about forecasting the future. Needless to say, a difficult task. However, past successes, especially by recommender systems, have shown that limited short-term predictions are often successful. Recommender systems can be found on services such as Netflix or Amazon.
Prescriptive Analytics can be the most challenging and may require much subject matter expertise. Here, a solution is proposed to address a problem or issue. A data analyst can add new perspectives and help the SME evaluate possible scenarios.
Here are a few notes about the use of data analytics to ensure success.
- Business Goals: Identify your goals before engaging a data analyst. Projects that start out with find “something of interest” is like going down a rabbit hole.
- Start Simple: Firms with no experience with data analytics are better off beginning with an easier project where a short-term win is more likely.
- Data Preparation: Preparation can consume a lot of the project’s time. Estimates range from 50-80%. If you think your data is in relatively good shape because it is in a data base, you might be surprised to learn otherwise. Even with clean data, some analysis techniques require much data preparation.
- Dead Data or Wishful Thinking: If you think that there is no relationship in the data, you may very well be right. Don’t expect a data analyst to magically find something. Most contributions from research are done in small incremental steps.
- Think Outside of the Box: Radically new approaches should be contemplated when possible. It need not be quite as ingenious as the Mars Pathfinder inflatable airbag landing system but the use of tweets for TV audience share was quite good.
- Good Luck: NO! Luck is not a prerequisite. Good planning and expertise will help ensure your success.
In closing, I would be remiss if I didn’t mention the UW-Stevens Point data analytics program, which is patterned against some of the best data analytics and data science programs in the country. The UWSP program covers data preparation, machine learning, data mining, predictive analytics, big data analytics, statistics, R & Python programming, and database. Out of 120 credits required for college graduation, 70 of those credits are dedicated to data analytics; a very in depth major.
Our program has faculty who not only have academic experience, but also are industry experts in data science, market research and computer science. Our product is our students and we are proud of what they represent. We have heard and seen from employers how our students are able to make contributions quite quickly to these organization.
If you have any questions or would like to reach out, please contact, Kurt Pflughoeft, Sentry Endowed Chair of Computational Analytics and Director of the Center for Data Analytics. The center offers data analytic services to businesses through faculty and students.